Kraude, R.
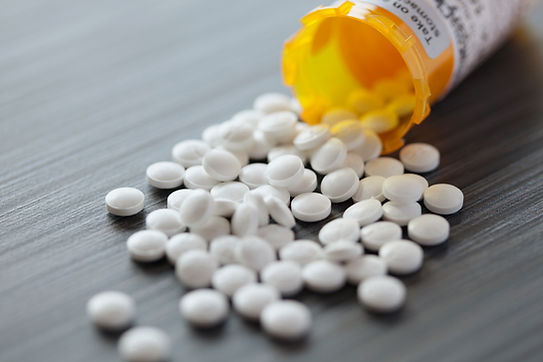
Failure is expected when developing new pharmaceutical products because it is a complex process with high failure rates. Rather than simply trying to avoid failure, organizations seek to ensure failure happens quickly because resources are limited and development costs are a function of time. In this study, we investigate the drivers of drug development failure rate to assist in reducing the risk of the drug development process. We construct an archival dataset of 8746 drug development projects drawn from three separate sources: Medtrack, the NIH clinical trials database and the USPTO patent database. We run a random survival forest machine learning algorithm to predict time to failure. The most important variables in predicting failure rates varied between the three clinical trial phases, highlighting the balance between strategy and environment. Additionally, we fit a Weibull proportional hazards survival model on the full data to translate the predictions into failure rates.
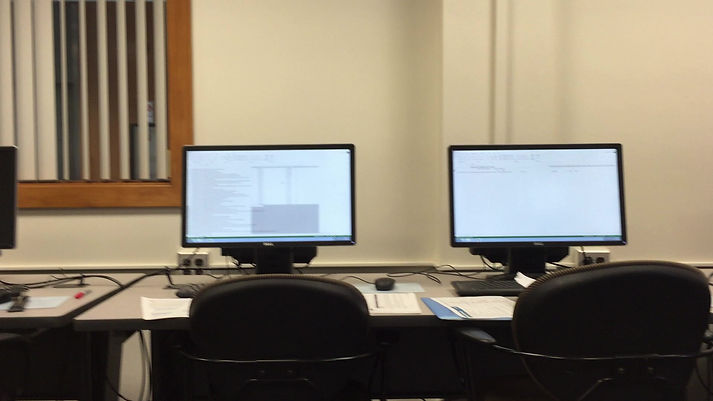